In recent years, we have always published open access and communicate what we do in the media. But during this coming academic year we will start a new, much more open way of communicating what we do.
Instead of basing our research solely around a series of academic papers, we will use the new collective-behavior website to communicate our results before, during and after they are published. We will also communicate smaller, interesting results that may never make it in to published papers but are central to debates in science and society. We will do interactive science, where we take in suggestions about what we should work on and provide rapid results.
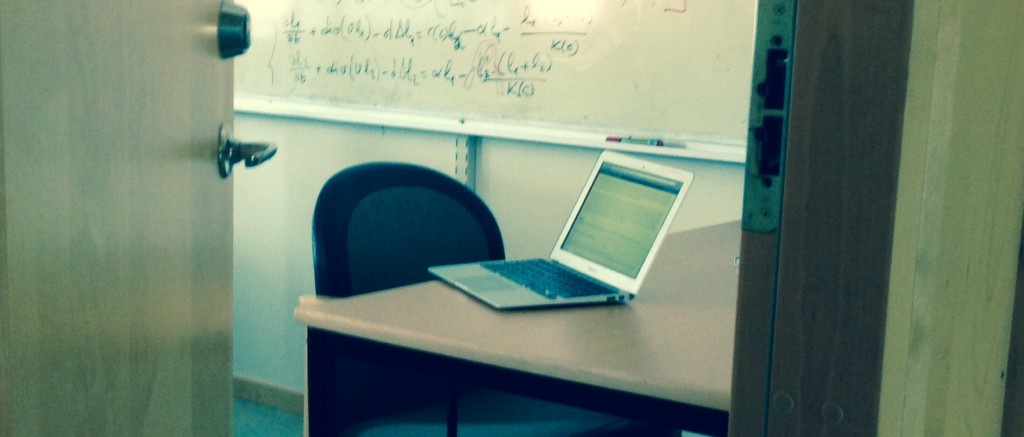
The challenge will be to improve speed and accessibility of communication without reducing quality. Our answer is to write 500-1500 word analysis pieces where we use our skills as mathematical modellers and scientists to answer specific questions. Our aim is to reach much more widely than our current research circle. For example, one of the first articles on the new webpage is an analysis of pop charts using a gravity model. This is a fun application of our research, illustrating how the methods we use can shed light on the every day phenomena of popularity. Another example that will appear soon is a Turing Test for fish schools: can you tell the difference between a simulated fish school and a real one? As well as the fun-side of research we will deal with important issues in society. For example, in an upcoming piece, we extend on empirical work on segregation in biology departments, using a model to understand what we can do to improve equality in universities.
Other web articles will directly extend our published work, such as a post today on how countries across the world have become more emancipated. We will of course continue to publish regular updates on what we are up to in our usual research. The articles we write will use the same scientific standards as we use in all our research, but with less literature background and more concise, clear language. We will communicate ideas, rapidly and clearly, so as to be useful both to other researchers and the general public. We aim to write to a high scientific standard and refer to peer reviewed articles. That said, we encourage you as a reader to put our work in a wider context, both through following the links we give and by consulting other parts of the literature.
The change starts now, and over the next few weeks more articles will start appearing on our www.collective-behavior.com. So welcome to our own version of Open Science 4.0!
This is a copy post and can also be read on the site itself.